
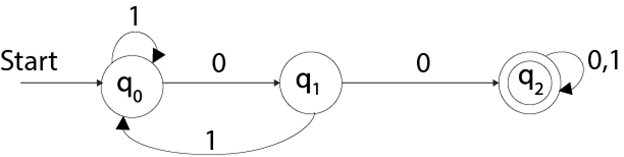
Meanwhile, the growing field of Deep Learning drew from HMM model-fitting algorithms to train Recurrent Neural Networks (RNNs) with sequential inputs and outputs specifically, using Connectionist Temporal Classification (CTC) which is based on the Forward-Backward algorithm ( Graves et al., 2006). HMMs (and automata more generally) continue to represent the state of the art for many bioinformatic tasks for example, when reconstructing the indel histories of ancestral sequences ( Holmes, 2017 Löytynoja and Goldman, 2005 Westesson et al., 2012) or aligning protein to DNA ( Birney et al., 2004). Further evolution of these ideas including HMMs for multiple sequence alignment ( Do et al., 2005 Holmes and Bruno, 2001), comparative genefinding ( Alexandersson et al., 2003 Meyer and Durbin, 2004) and phylogenetics ( Siepel and Haussler, 2003 Siepel et al., 2006 Suchard and Redelings, 2006) occurred in the 2000s. This inspired new applications of HMMs in emerging areas of sequence analysis, such as computational gene prediction ( Burge and Karlin, 1997).

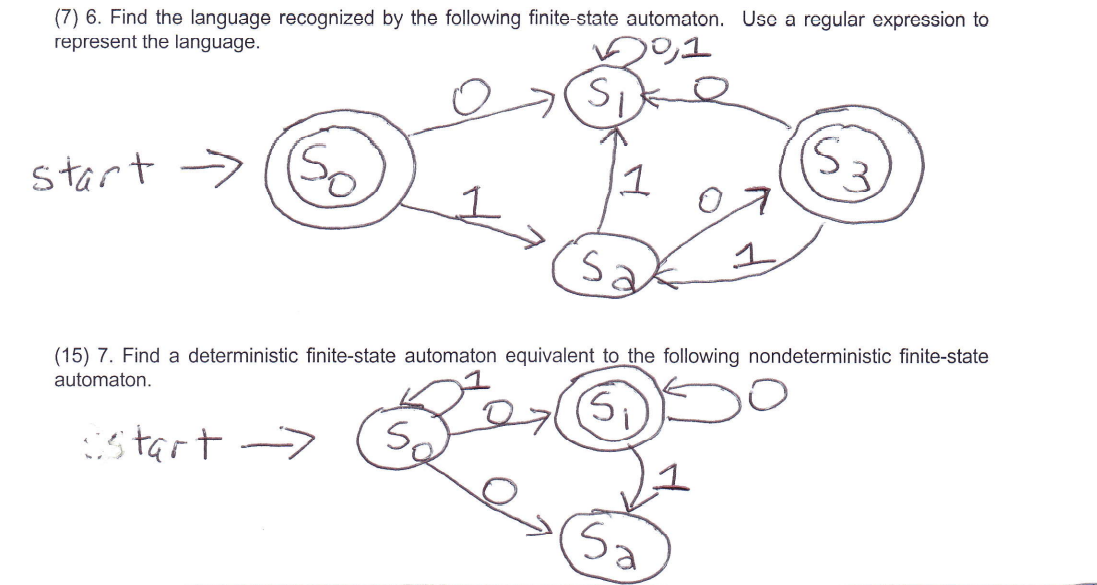
The 1990s saw the probabilistic interpretation of both these kinds of machine as Hidden Markov Models (HMMs) respectively, the ‘pair HMM’ and the ‘profile HMM’ ( Brown et al., 1993 Durbin et al., 1998). Gribskov’s protein profiles of the late 1980s are state machines too ( Gribskov et al., 1987). The venerable Needleman–Wunsch, Smith–Waterman and Gotoh algorithms from the 1970s and early 1980s can be thought of as aligning pairs of sequences to input–output automata ( Gotoh, 1982 Needleman and Wunsch, 1970 Smith and Waterman, 1981). Bioinformatics is a field littered with state machines, many of them still functional.
